Get more wins: Experimentation metrics for program success

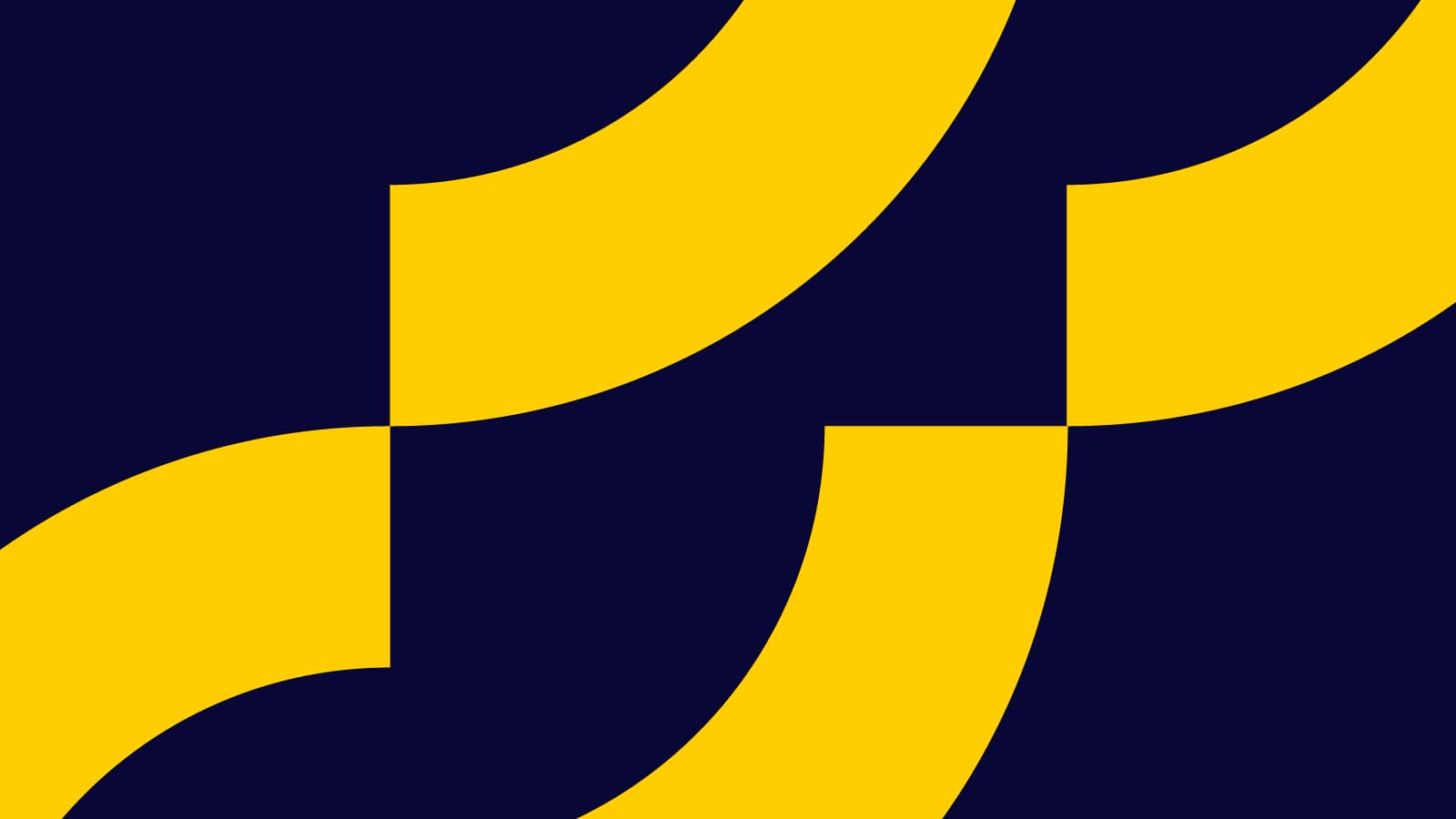
As we mentioned in our first post in this series, many companies have realized that optimization of their digital products and experiences is no longer a negotiable aspect of their digital strategy. Optimizely’s Strategy and Value team often fields questions on how to operationalize a testing program and how to choose an executive sponsor, but for those new to experimentation, the most important unknown might be how to justify and illustrate the ROI of an experimentation platform and optimization program. There are many methods of quantifying the ROI of your program, but there are two fundamental sets of metrics you can focus on to help build your case: your company’s key metrics and the metrics of your program.
Your Company’s Key Goals
As you build out an optimization program at your company, it’s important to align your program’s testing efforts to your company’s key goals. By focusing your experimentation program on influencing key metrics, you ensure that your program is having a direct and attributable influence on the most important KPIs for your business – this will absolutely help you with subsequent ROI conversations. In the illustration below, you’ll find a goal tree, which is a visual diagram of how an overarching company goal (Revenue) trickles down to Experiment Goals that you can influence through your testing. If you’re not familiar with goal trees, you can find a great explanation in Optimizely’s Knowledge Base, as well as industry-specific goal tree templates to help you get started.
Goal trees are multi-purpose: they help you map out the most valuable metrics for your business and encourage focusing your optimization efforts on areas where wins will generate more visibility and traction. Goal trees also provide a great conversation starter to prompt discussion and internal alignment. This isn’t a one-and-done exercise – it’s really important to level set and revisit these goals with your executive sponsor and key leadership, especially as directions and initiatives change. A quick example: in the last two years, a ton of OTT & SVOD streaming brands have launched, initially focusing on customer acquisition metrics like free trial signups, free trial conversion rate, and total subscribers. However, as COVID restrictions lighten up and users reevaluate their entertainment spending, these same brands might shift focus to customer engagement & retention metrics, like total minutes watched, streaming starts, and monthly active logins – those may become the key business metrics for their executives, stakeholders, and stockholders.
Once you’re aligned on your company goals and the key metrics you want to influence, you’ll want to focus on running conclusive, winning experiments. This leads us to the next set of metrics you want to measure and regularly report on: the metrics of your Experimentation Program.
Your Experimentation Program’s Metrics
Many of us have Apple Watches or Fitbits to keep us on track and mindful of our daily behavior – steps taken, calories burned, hours slept, etc. In many ways, your Experimentation Program’s metrics are your program’s Fitbit – they show your program’s health, growth, and where you can improve. First, we’ll walk through the primary metrics that directly affect getting more wins, and then we’ll dive deeper into ancillary metrics that can help you build a holistic view of your program’s health.
Three Key Metrics
Velocity – the number of tests you are running per month, quarter, year
Velocity is probably the most standard “health check” metric, as it can easily show the growth in your program and can often help you advocate for additional resources. As a benchmark, from 2019 – 2020, Optimizely customers’ velocity increased by about 7% on average (including usage of any part of our suite of products), so you’ll want to aim for a minimum of +10-20% year over year growth in your number of tests. For context, many of our clients are aiming to double their number of tests (+100%) year over year, but this level of growth is dependent on your program’s resources and maturity. One callout: some programs include tests and test ideas in development as part of their velocity, so you’ll want to decide in your reporting if you are including those as well versus only tests launched. If you’re struggling to gain velocity, many Optimizely customers utilize our On-Demand Services offering to scale up their monthly test number.
Conclusive Rate: The % of experiments that have hit a statistically significant result on the primary metric, whether it’s a win or a loss.
You can increase your velocity all you want, but if you aren’t getting conclusive results, those tests aren’t showing you anything you can action on. You can learn as much from the losses as the wins, and in fact, sometimes the losses save you serious money by preventing the roll out of a feature that would have tanked your metrics. On average, Optimizely clients have a conclusive rate of around 35-40%, meaning 4 of every 10 tests are conclusive. If you’re below 33%, you might want to check into how you’re setting up your tests or take bigger risks in your variations, as a test can’t potentially be a win if it’s not conclusive!
Win Rate – the % of experiments that have hit a statistically significant result and uplift on the primary metric.
This metric is important because even though you learn from all conclusive tests, the wins on revenue are the ones that can easily be attributed to a future ROI conversation. As a benchmark, Optimizely clients on average have a win rate of around 20% for all experiments, but a win rate of only 10% for experiments tied to revenue. So that also helps explain why you need to run more tests and have them be conclusive – it’s a cyclical thing!
More Metrics that Matter
While those are the three metrics you hear experimentation practitioners mention most frequently, there are additional metrics that can be incredibly helpful in identifying opportunities or diagnosing potential issues within your program.
- Development time – how long is it taking for tests to be built and launched?
If you’re struggling to increase your velocity, looking into your dev time can help you identify where you are getting held up in your testing cycle and where you might need more resources. - Time to Production – average days it takes to launch a winning variation
Getting wins is great, but they’re only true wins when they are live on your site in production. If you are struggling to get those wins on your roadmap or having to regularly run winning variations at 100% through your testing platform, perhaps you need to include your product or IT teams in your monthly meetings and let them know what tests you’ll be running. Maybe your Exec Sponsor can grease the wheels a bit? Another option: investigate a way to do this faster and without IT resources, like making the changes yourself within your CMS. - Variations – how many variations are we running per test on average?
Back in the day, many of us called this practice “A/B testing,” and it was often just that: A vs B. Now, we have the research and proof that tests with more variations (particularly 4 or more) win at a much higher rate, as well as tests with more lines of code. So, don’t sacrifice complexity just to get tests out the door – it’s truly an art and a science of trying to grow your velocity while also running complex, well-thought out, and bold experiments.
Now that you know the players you need on the court and the metrics you need to influence, we’ll use our next blog post to cover some key pieces for operationalizing your program. If you want to dig into any of the topics further, please find links below!
Interested in more content about goal trees & metrics?
Optimizely’s Knowledge Base has great articles on goal trees, as well as on primary and secondary metrics. Alek Toumert does a great walk through of output and input metrics, and Jon Noronha explains how he chose metrics while at Microsoft. And if you’re struggling to define your North Star Metric, check out this blog post or this article for more guidance on that topic.
Interested in more articles about program metrics?
There’s a lot of great content on Knowledge Base around Taking Action Based on Experiment Results, Statistical Significance, & Confidence Intervals. Alek talks about Revenue as a Primary Metric, and Jon Noronha has another awesome blog post about scaling velocity and quality. You might see Program Maturity mentioned across these articles – find out your program’s maturity level by taking our Maturity Model quiz.